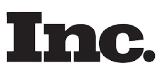
Turned Down for a Business Loan? A.I. Could Be to Blame
How entrepreneurs can avoid getting their credit application caught in a machine learning trap.
The frosty credit environment that small businesses have been experiencing for the past year could be getting even chillier. That’s all thanks to artificial intelligence.
Financial institutions are increasingly employing A.I. and machine learning, including for work like risk assessment and credit scoring. A survey conducted in June by the mortgage industry advisory firm Stratmor Group found that 22 percent of lenders are already using A.I., up from 13 percent in 2018. For lenders, particularly smaller ones strapped for staff and resources, investing in automation has the potential to add up to major cost savings. By 2030, those savings could reach as high as $1 trillion for the entire industry, according to a report from the financial research firm Autonomous, which found that banks could cut costs by 22 percent by using artificial intelligence.
While A.I. could be a major boon for lenders, which now have the ability to gather and process much more consumer information than the standard debt-to-income ratio, the federal government is considering what that means for borrowers, particularly those who find themselves turned down for a loan or credit line. Last month, the Consumer Financial Protection Bureau issued new guidance about legal requirements for lenders using artificial intelligence and other advanced algorithms. The agency said that if a borrower is denied credit, the lender must provide “specific and accurate reasons” why that decision was made.
“Artificial intelligence is expanding the data used for lending decisions, and also growing the list of potential reasons for why credit is denied,” said CFPB director Rohit Chopra in a statement. “Creditors must be able to specifically explain their reasons for denial.”
Richard Gusmano, the founder and CEO of Business Credit Consultants, which helps small businesses obtain credit, says that the CFPB is trying to safeguard against machine learning becoming the sole decision maker determining which small businesses are approved for a loan and which ones are denied. Gusmano sees the rule as a positive one for businesses but stresses that the guidance was “such a broad stroke” that questions remain about how it will be implemented.
“We’re not seeing a timeline,” says Gusmano. “We need reasons, but how quickly will those reasons come out? … That’s the thing that I’m most interested to see.”
The best defense against having your loan application rejected by A.I. is to be even more scrupulous than usual with your documentation. “A lot of the information that banks are given or a lot of information that accountants put on the top form of the tax return is inaccurate,” says Gusmano, who adds that this problem will be most acute for businesses working with a lender for the first time.
In his experience, one of the most common mistakes is a company’s North American Industry Classification System, or NAICS, code, which the federal government uses to classify businesses by sector. The six-digit number is often chosen by the accountant filing a business’s taxes, so owners should check that the category is accurate. Talking with your accountant before applying for credit is also important, he says, because their objectives when preparing tax documents are often in direct confrontation with the determinants that A.I. models are trained to look for in creditworthy businesses.
“Accountants want to show losses to mitigate tax,” says Gusmano. “Let’s make sure Mr. or Mrs. Accountant is not being overly aggressive with losses, and let’s make sure that the numbers make sense.”
The pandemic, Gusmano says, has also created unique problems for businesses trying to circumvent banks’ algorithmic models. Some of his clients who have transitioned to a remote model or moved offices in the past few years have been tripped up by inconsistent business addresses.
“It’s common-sense stuff, but there are mistakes all over the map,” he says.